Neuroscience inspired the early design of artificial intelligence systems, and the two fields have a long history of collaboration and reciprocity. With the rapid progress in AI in recent years, driven by innovative engineering, the mutual influence of the two fields has evolved.
Neuroscientists, computer scientists, and engineers from a variety of institutions, including Stanford University and MIT, shared recent research developments in neuroscience and AI and discussed the apparent divergence of the two fields as well as continued opportunities for interdisciplinary collaboration and innovation at the NeuroAI 2024 workshop hosted by the Center for Cognitive Neuroscience and the Breaking the Neural Code Cluster last week at the Hanover Inn.
“The center’s annual workshop is a great opportunity to bring world-class researchers to Dartmouth and infuse ideas and discussion into our community,” said Diana L. Taylor Distinguished Professor in Neuroscience Tor Wager, who co-organized the Oct. 21-22 workshop with other Arts and Sciences faculty members.
“In some sense, there’s no field closer to AI than neuroscience. The principles underlying artificial intelligence are directly inspired by or adapted from principles of brain function. There has been a lot of cross talk, and over the past decade, this has accelerated again,” said Wager.
The workshop drew over 140 attendees—undergraduate and graduate students and faculty members—from across schools and departments at Dartmouth, including the Department of Psychological and Brain Sciences, the Department of Computer Science, the Department of Mathematics, the Geisel School of Medicine, the Guarini School of Graduate and Advanced Studies, and Thayer School of Engineering.
Across four sessions spanning two days, speakers from Dartmouth and other universities introduced the audience to cutting-edge AI models that are optimized to perform higher level cognitive operations such as deciphering human speech amidst a cacophony of background noise, demonstrating improved vision and language performance, and recognizing social interactions.
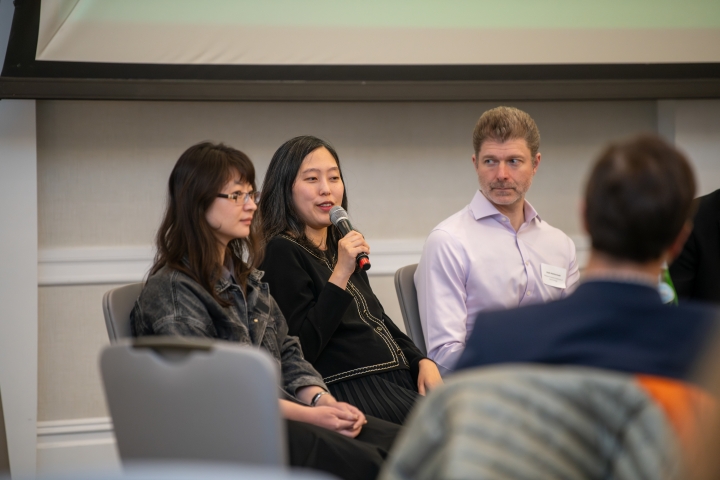
Yujun Yan, assistant professor of computer science, proposed strategies to improve the estimation of brain graphs that model the complex functional relationships among brain regions and their relationships with human emotion and cognition.
Souyoung Jin, assistant professor of computer science, presented a new approach to create models that can understand video content. These models can be used for generating video captions, summarizing content in videos, and generating detailed audio descriptions for people with visual impairments.
Panel discussions at the end of each session provided an opportunity for the audience to interact with speakers and opened the floor to discussion.
In particular, the question of whether AI continues to draw inspiration from neuroscience was revisited several times throughout the workshop. For some, the fields appear to have diverged, at least in the short-term, as AI researchers are engaged in building more powerful and sophisticated models by scaling up complex neural network architectures.
On the other hand, some researchers believe that ideas based on biological principles could enable researchers to revolutionize AI by developing models that are powerful, yet more efficient both in terms of the amount of data required to train them and the energy they consume.
“There isn’t one answer, and I think it is healthy to have a diversity of opinions,” said Wager.
The workshop covered many different layers of the depth of AI usage, from machine learning models that are used to analyze data to future directions in AI, said Byeol Kim, a PhD student in the psychological and brain sciences department.
“I love the kind of momentum such events bring, encouraging all of us students and researchers to learn and embrace new technologies,” said Kim. “It’s quite insightful and interesting to hear the thoughts and perspectives of researchers from different backgrounds.”